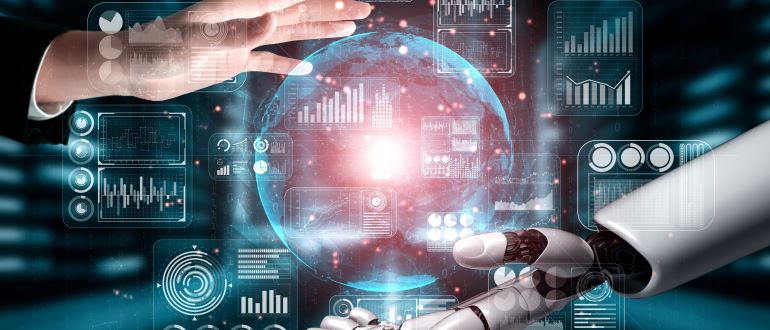
A team of a physicists at RIKEN, a Japanese research organization, have developed a method to use AI to improve error correction in quantum computing, an advance that supports quantum computing’s growth toward mainstream adoption.
Many industry experts have theorized about the ways that the supercomputing power of quantum computing can support AI. In a fresh twist, the RIKEN research team turned this idea around to develop a technique for AI to support quantum. To do so, they focused on one of the thorniest challenges in quantum computing, the stability of qubits.
In contrast with the bits used in traditional computing, which can only be a 1 or 0, the qubits in quantum can exist in a superposition of both states simultaneously. This ability to hold superposition provides quantum with its ability to far surpass the processing power of traditional computers. But qubits are hyper-sensitive to even minor environmental disturbances—including electromagnetic interference—which corrupts the information being processed and creates errors.
One long-established technique to correct these errors is to use the Gottesman–Kitaev–Preskill (GKP) code, which helps encode the quantum in a reliably oscillating state. Among the benefits of GKP is that it assists error correction in quantum systems without requiring a great deal of hardware to support it.
GKP encoding systems, however, rely on so-called squeezed states; squeezed states of light have noise below the standard quantum limit, which enables ultra-precise measurements. These states are optimal but they are difficult to generate and control. Getting GKP codes to work in real world applications has been a hurdle holding back quantum’s evolution.
The RIKEN team developed a technique to leverage deep learning to optimize GKP states. In a paper published in the journal Physical Review of Letters, the team laid out its methodology for reducing the need to employ large-amplitude squeezed states and at the same time maintaining the GKP codes’ error correction properties. The RIKEN researchers found that a neural network produced a more efficient encoding method than they hypothesized.
GKP codes optimized with this technique “outperform the best conventional ones, requiring fewer squeezed coherent states, while maintaining simple and generalized stabilizer operators,” the team wrote in its paper. Furthermore, their new method performed better than earlier methods “with just one third of the number of squeezed coherent states at a squeezing level of 9.55 dB. This optimization drastically decreases the complexity of codewords while improving error correctability.”
The team’s findings help quantum evolve toward fault-tolerant quantum systems that are scalable enough to support mainstream adoption.
“By leveraging AI, our method fine-tunes the structure of GKP states, striking an optimal balance between resource efficiency and error resilience,” said Yexiong Zeng, one of the researchers.
The RIKEN team cautioned that although its development in deep learning supported GKP encoding on some quantum platforms, it might not be suited for every quantum scenario. Still, the team is encouraged, and is now planning to further develop its work on GKP codes to help scale multi-logical systems, which involves dividing a single computing device into a multiple entities.