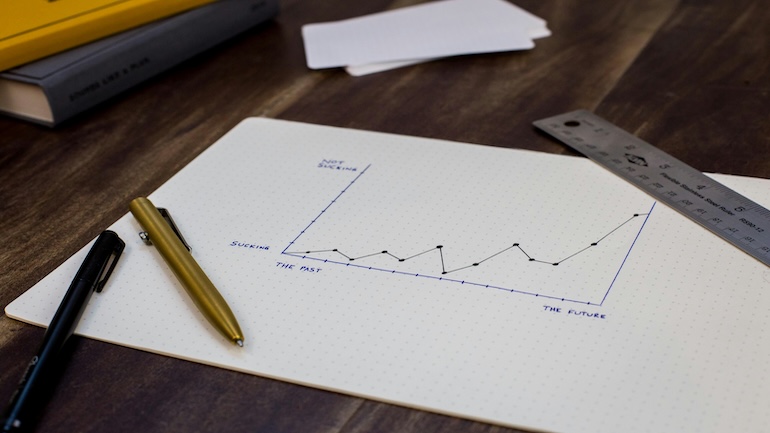
In today’s digital landscape, organizations are investing heavily in advanced software solutions and artificial intelligence (AI) capabilities to drive innovation and competitive advantage. Yet many are finding these investments fail to deliver expected returns.
The culprit? Data silos. Data silos fragment critical information across the enterprise, creating invisible barriers to innovation. In fact, poor data quality costs organizations $12.9 million a year on average at the low end, says Gartner research. More than two-thirds (68%) of CDOs said data quality was their top challenge, according to the Ataccama Data Trust Report 2025. Meanwhile, four-in-ten struggle to maintain consistent data quality, which directly hinders AI outcomes.
The Hidden Innovation Blocker
Despite significant advancements in software development methodologies, cloud platforms and AI technologies, many organizations struggle to achieve breakthrough innovation. The fundamental issue often lies not with the technologies themselves, but with how data is structured, accessed and shared across the enterprise.
Data silos create formidable barriers to innovation in several critical ways:
- Limited Visibility: When critical data remains trapped in departmental silos, developers and architects lack the comprehensive view needed to identify opportunities for innovation. A partial picture leads to partial solutions.
- Duplicated Efforts: Teams working with siloed data often unknowingly replicate work being done elsewhere, wasting resources that could be directed toward innovation.
- Inconsistent Data Models: Siloed systems typically implement different data models and taxonomies, making integration increasingly complex and forcing technical teams to focus on baseline connectivity rather than value creation.
- Innovation Inertia: The organizational friction created by data silos means new initiatives require disproportionate effort just to access necessary data, creating resistance to experimentation and innovation.
Real-World Impact: The Insurance Innovation Gap
Historically, insurers have suffered from poor data quality, primarily caused by having vast amounts of unstructured data stored in a plethora of systems, according to Moodyโs.
Consider a mid-sized insurance company that invested heavily in AI-powered underwriting tools to accelerate policy approvals. Despite significant technology investments, their innovation initiative stalled because of entrenched data silos.
The customer information system maintained by the sales department contained prospect details and initial application data. The underwriting department used a specialized risk assessment system with its own customer profiles. Meanwhile, the claims department operated yet another system with critical loss history data. Each department effectively “owned” their slice of the customer data, creating multiple disconnected views of the same policyholders.
When the company attempted to implement AI-based underwriting, it discovered the models required seamless access to all three data sources to make accurate risk assessments. The technical team spent nine months building custom integrations between systems, only to find that data inconsistencies between silos made the AI results unreliable. Their innovation initiative โ which promised 48-hour policy approvals โ was reduced to a limited pilot program that couldn’t scale.ย
First-Mile Data: The Innovation Starting Point
The insurance company’s challenges were particularly acute with first-mile data โ the varied and often unstructured information from external sources like broker submissions, customer applications and third-party risk reports. This “first-mile data problem” created a bottleneck at the very beginning of its innovation pipeline.
After recognizing this fundamental barrier, the company shifted focus. Rather than continuing to invest in advanced AI models, it implemented an intelligent data automation platform that addressed its first-mile data challenges by:
- Creating standardized data models that spanned departmental boundaries
- Automating the ingestion and transformation of external data from multiple sources
- Establishing a unified customer data platform accessible to all systems
Within three months of this approach, the previously stalled AI underwriting initiative was processing simple policies automatically, and within six months achieved its original goal of 48-hour approvals for 70% of applications.
Breaking Down the Barriers
Addressing data silos requires both technological and organizational approaches:
- API-First Thinking: Implementing API-first architectures ensures systems are designed for connectivity from the ground up rather than treating integration as an afterthought.
- Data Mesh Implementation: Consider data mesh approaches that distribute data ownership while maintaining governance, empowering teams to both contribute to and benefit from enterprise data assets.
- Cross-Functional Data Teams: Form teams that bring together business and technical stakeholders to identify how data sharing can enable innovation and establish appropriate governance.
- Intelligent Data Automation: Modern platforms that automate the process of ingesting, transforming and standardizing diverse data sources can dramatically reduce the technical barriers to data sharing.
- Data Catalogs: Implement enterprise data catalogs that make data discoverable across the organization, enabling teams to identify and leverage relevant information regardless of where it resides.
As technology leaders, we must recognize that software innovation isn’t just about adopting the latest technologies โ it’s about ensuring data can flow freely to where it creates the most value. This requires deliberate effort to identify and eliminate data silos through both technical and organizational means.
By addressing these barriers, organizations can ensure their investments in advanced technologies deliver their full potential rather than simply adding new capabilities on top of fragmented foundations. In an era where data-driven innovation increasingly determines competitive success, breaking down data silos isn’t just a technical challenge โ it’s a strategic imperative.