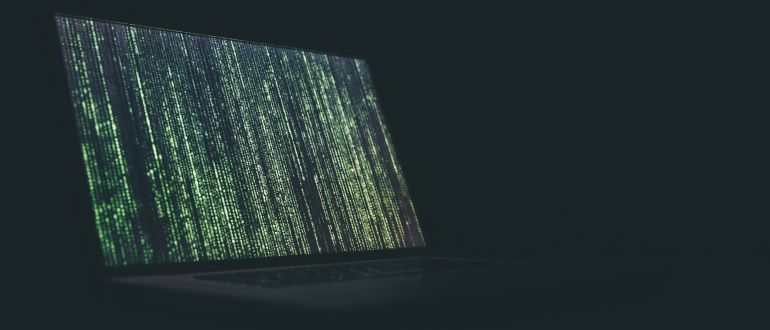
Artificial intelligence (AI) is reshaping IT service management (ITSM), offering the potential to automate processes, enhance decision-making and improve efficiency. But for many organizations, the biggest roadblock isn’t AI itself—it’s their data.
Too often, IT teams dive into AI projects only to realize their data isn’t ready. Inconsistent formatting, missing pieces and poor structure can derail even the most promising initiatives. Without high-quality, well-prepared data, AI can’t deliver the insights and automation organizations expect.
Let’s review how IT and data leaders can lay the groundwork for AI success by focusing on data readiness, organizational resources and key success metrics.
The Data Challenge: AI Needs Clean, Usable Data
Think of AI as a high-performance sports car; without quality fuel, it won’t go far.
Many organizations face common data challenges that prevent AI from running at full speed. This includes:
- Scattered, incomplete, or siloed data: AI models need comprehensive datasets to generate accurate results. If key information is missing, predictions will be unreliable.
- Data quality issues: Inconsistencies, errors and lack of standardization can throw off AI models and lead to flawed decision-making.
- Lack of training data: AI models need properly labeled data to learn from. Without it, they won’t function effectively.
To fix these issues, IT teams should start with a comprehensive data audit. This means assessing whether data is clean, complete and structured enough to support AI initiatives. If there are gaps, solutions like data tagging services or normalization strategies can help standardize datasets and improve AI outcomes.
Readiness Checklist: Is Your Organization AI-Ready?
Before rolling out AI, IT leaders need to evaluate whether their organization is truly prepared. Here are three critical areas to assess:
1. Key Metrics and Business Alignment
- AI should align with business goals—so first, define the key performance indicators (KPIs) that matter most.
- Track pre-AI metrics like error rates, resolution times, transaction volumes and quality scores to establish a baseline.
- Conduct a gap analysis to compare current operations to the ideal AI-enhanced future state. This will help identify where AI can make the biggest impact.
2. Data Quality and Availability
- AI depends on data that is accurate, well-organized and extensive enough for training models.
- IT teams must assess whether their existing datasets are structured and consistent across all systems.
- If data is scattered or inconsistent, investing in data normalization and integration will be essential before moving forward.
3. Infrastructure and Talent
- Do you have the right people to manage AI initiatives? If not, consider upskilling teams or partnering with external AI specialists.
- While AI often requires in-house expertise, cloud-based AI tools from major providers (AWS, Google, Microsoft, etc.) offer accessible solutions with built-in algorithms.
- Ensure IT infrastructure can handle AI workloads, including scalability, security and storage capacity.
3. Measuring AI Success: Did It Deliver Real Value?
AI should ultimately move the needle on key business goals. To measure success, IT leaders should track:
- Accuracy and Impact: Did the AI system deliver reliable, high-quality results? Were data quality issues a limiting factor?
- Operational Performance: Was the project completed on time? Did AI meet expectations in streamlining workflows or automating tasks?
- Business Outcomes: Did AI reduce manual workloads, improve efficiency, or enhance customer experience?
- Data Maturity Improvements: Did the project help resolve past data challenges and pave the way for future AI initiatives?
Regular performance monitoring and feedback loops will help IT teams refine AI applications over time, ensuring they continuously align with evolving business needs.
Laying the Foundation for AI-Driven ITSM
AI in ITSM holds tremendous promise—but only if the right groundwork is in place. Organizations that prioritize data quality, infrastructure readiness and measurable success indicators will be the ones that truly benefit from AI-driven automation and decision-making.
At the end of the day, AI isn’t just about adopting new technology—it’s about ensuring that your data, processes and infrastructure are built to support it.
By taking the time to prepare, IT leaders can position their organizations to unlock the full potential of AI in ITSM, transforming both efficiency and innovation for years to come.